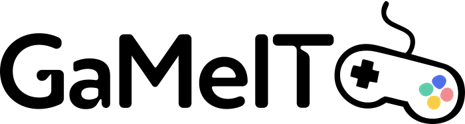
GaMeIT: Data-driven Gamification to Improve Quality in Medical Image Annotation Tasks
- Contact:
Ali Sunyaev
- Project Group:
Ali Sunyaev, Manuel Schmidt-Kraepelin, Scott Thiebes, Simon Warsinsky
- Funding:
Helmholtz Association
- Partner:
University Hospital Heidelberg
- Startdate:
01.07.2021
- Enddate:
30.06.2024
Cognitive surgical assistance systems, such as surgical robots require image-based scene understanding to perceive the surgery context, comprehend the surgery procedure, and eventually generate safe trajectories to assist during the surgery. To achieve such scene understanding, recognition and semantic segmentation of different surgery aspects (e.g., shown organs, used surgical tools, different surgery stages) are necessary pre-conditions. Machine Learning (ML) approaches are a promising technology for semantic segmentation of images. To train robots with ML methods, annotated image data (e.g. in the form of videos) is required. Image annotation of surgical images and videos is often manually conducted by healthcare professionals. This is necessary since a certain level of medical expertise is required. The process of manual annotation is prone to human errors since it can be tedious, monotonous, and exhausting. As a consequence, poor label quality is a common problem. However, for surgical robots to improve surgical procedures, sufficient data quality of annotated images is a decisive factor. If ML models for surgical robots are trained based on poorly labeled image data, this may negatively influence patients’ health since the robots cannot be utilized to their full potential. In this project, we address the problem of poor label quality of surgical image data by augmenting the annotation process with persuasive technology. In particular, we design, implement, and evaluate a data driven ML-based gamification concept to foster annotators engagement and, thereby, ensure high quality data labeling. By drawing on ML methods, the gamification concept is able to adapt to individual user preferences and overcome the weaknesses of one-size-fits-all gamification approaches.